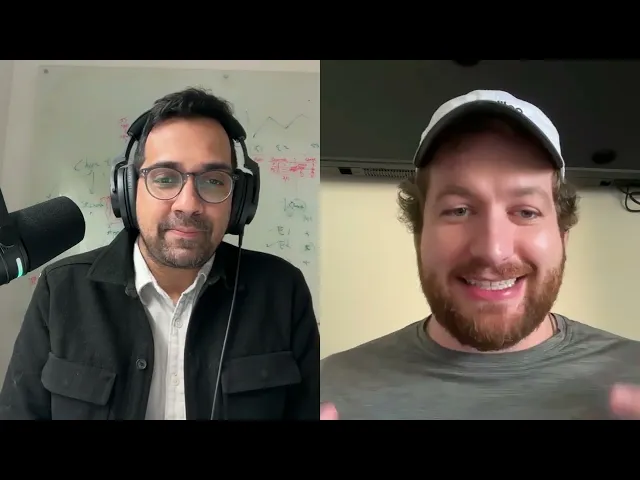
Feb 24, 2025
Understanding and Evaluating AI Agentic Systems


Conor Bronsdon
Head of Developer Awareness
Conor Bronsdon
Head of Developer Awareness


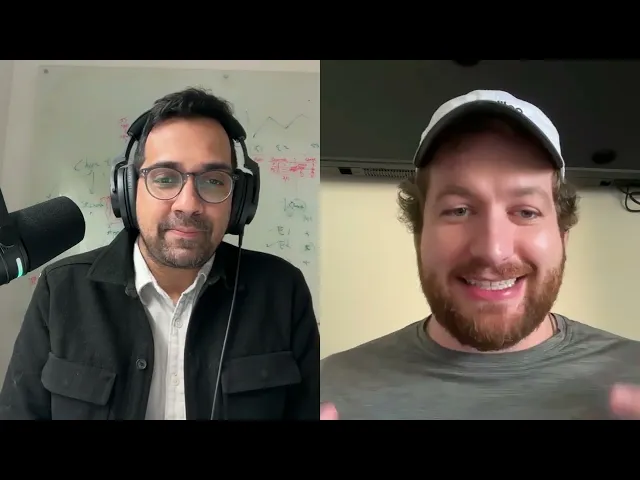
AI's agentic systems are making waves. Even Microsoft's Satya Nadella has shifted from talking about Software as a Service to "agents as a service." This isn't a minor update—it's reshaping how AI works across industries, opening doors to smarter automation and independent operations.
In a Chain of Thought podcast episode, Conor Bronsdon, Head of Developer Awareness at Galileo, and Atindriyo Sanyal, Galileo's CTO, explored agentic systems. They highlighted real-world impact, especially in customer service, where digital agents function as virtual team members.
Sanyal described how these systems combine large language model calls, database lookups, and more. "Agents get a little bit more complicated with additional components, which are stitched together as a directed acyclic graph or a DAG," he said. This architecture enables more intelligent interactions beyond simple Q&A.
The Structure and Functionality of Agentic Systems
Agentic systems represent a significant AI advancement. Unlike traditional single-task systems, they integrate multiple components to work independently. This evolution mirrors developments in Large Language Models (LLMs), databases, and APIs.

Key Components
As Sanyal explains, agentic systems have "a bunch of extra components that... get a little bit more complicated in terms of there's additional components, which are stitched together as, like, a directed acyclic graph or a DAG."
The directed acyclic graph structure is crucial to agentic systems, allowing sequential node operations—each potentially an LLM call, database lookup, or tool operation. This enables the system to function like a digital employee, handling tasks autonomously.
At their core, these systems rely on prompt engineering that guides the decision-making process. These prompts act as instruction sets, helping determine when to retrieve information from external sources versus generating responses from internal knowledge.
Memory components enable agents to recall past interactions and maintain context throughout complex conversations. This persistent memory creates more natural, human-like exchanges and allows for relationship-building between the AI and its users.
API integrations serve as the agent's connection to the external world, allowing it to perform actions like booking appointments, processing payments, or updating records in real-time. These capabilities transform agents from passive responders to active participants in business workflows.
Comparison With Traditional AI Systems
The design and purpose differences between traditional AI and agentic systems are stark. Traditional AI typically handles specific tasks with defined inputs and outputs, while agentic systems are built to mimic human decision-making across multiple tasks.
Traditional AI might handle one function at a time, but agentic systems combine several capabilities to reason and learn continuously, as highlighted in this AI frameworks comparison. This interconnection through the DAG structure helps these systems tackle complex challenges effectively. The choice between single vs multi-agent systems further influences their adaptability and performance.
Autonomy represents perhaps the most significant distinction. Traditional AI requires explicit human guidance for each new task, while agentic systems can determine the next steps independently based on context and goals, making them more versatile in unpredictable scenarios.
The cognitive architecture of agentic systems introduces a planning layer that allows for goal decomposition and prioritization. This enables agents to break complex objectives into manageable sub-tasks, much like a human professional would approach a multifaceted project.
Real-World Applications
Agentic systems show tremendous potential where automation and efficiency matter. For example, in financial services, they enhance customer service through chatbots that function as financial advisors, analyzing complex data for personalized guidance.
Bronsdon and Sanyal discussed the potential of a financial chatbot, asking, " Is the user's goal being met?" This goal-checking ability defines agentic systems. They satisfy client needs while maintaining security and compliance, critical in banking and healthcare. These systems adapt to real-time data changes, providing robust solutions for complex business challenges through advanced AI agentic workflows.
Agentic systems represent a stride toward smarter, self-sufficient AI applications, giving businesses better automation management tools. As this technology grows, it will continue transforming industries and driving digital advancement.
Evaluating Agentic Systems Through Task Completion and Error Management
As AI agents become increasingly integrated into business operations, performance evaluation becomes critical for reliability and effectiveness.
Importance of Task Completion Metrics
Agentic systems are autonomous, intelligent agents handling complex tasks. They go beyond responding to commands by utilizing databases, function calls, and logical operations to achieve goals. That's why task completion metrics are essential for evaluation.
"A notion of task completion or objective achievement” should be “one of the first things" examined when evaluating these systems, notes Sanyal. Whether it's a financial chatbot guiding investments or an AI agent processing data, measuring goal achievement is crucial. These metrics determine if agents are performing as intended, demonstrating their value and effectiveness.
Task completion metrics reveal alignment between user intent and agent action, highlighting instances where agents misinterpret requests. By benchmarking AI agents and tracking completion rates across different query types, organizations can identify pattern-based weaknesses requiring targeted improvements.
The granularity of these metrics matters significantly, as they should measure both overall task success and the accuracy of individual steps within multi-stage processes. This dual-layer assessment helps pinpoint exactly where breakdowns occur in complex workflows.
Customer satisfaction correlates strongly with successful task completion, making these metrics valuable business indicators beyond technical performance. When agents consistently achieve user goals efficiently, they build trust and encourage continued system engagement.
Error Compounding and its Implications
A major evaluation challenge is handling multiplying errors. Unlike traditional software, where isolated errors can be identified and fixed, agentic system mistakes can propagate. As Sanyal explains, "One mistake in any part of this workflow can lead to massive errors downstream."
Consider an AI system performing data retrieval through logical reasoning—an error anywhere can cascade through the system, amplifying its effects. Understanding error relationships helps teams develop better remediation and prevention strategies, strengthening system robustness and reliability.
The temporal dimension of error compounding presents unique challenges, as some errors remain dormant until triggered by specific conditions. These latent errors can create sudden system breakdowns when least expected, making comprehensive testing across diverse scenarios essential.
Recovery mechanisms become crucial design elements in agentic systems, providing failsafes that prevent complete system collapse when errors occur. Well-designed agents incorporate self-correction protocols that detect inconsistencies and attempt remediation before errors cascade.
Error correlation analysis offers valuable insights into systemic weaknesses, revealing whether failures cluster around particular components or interaction patterns. This analysis guides architectural improvements that strengthen not just error handling but the foundational design of the agent itself.
Galileo's Approach to Agentic Evaluations
Galileo leads in AI evaluation, particularly for agentic systems. Galileo’s approach addresses the growing need for robust tools that ensure AI systems perform well and align with intended goals.
Comprehensive Evaluation Platform
The complexity of agentic systems often renders traditional evaluation methods insufficient. New strategies have emerged, including real-time tracking, custom metrics, and detailed performance analysis. "There's no one size fits all," says Sanyal, highlighting the need for tailored solutions. This customization is crucial because AI applications vary greatly in components and goals.
Galileo's platform excels with its customizable evaluation metrics tailored to diverse agentic system needs. Sanyal continued, "We literally allow you to do two things. Number one, create your own agentic metric, which you care about… and apply it to the parts of the agents that you deem relevant."
A financial chatbot might use vector stores for data retrieval, requiring metrics focused on accuracy and relevance. Customization extends beyond creating metrics to integrating them into existing workflows, allowing developers to define metrics from simple Python functions to complex applications across their AI systems.
This flexibility lets developers evaluate precisely what matters most. Whether tracking an agent's goal-seeking steps or analyzing output accuracy, these custom metrics provide detailed performance insights. Monitoring tools like task completion metrics and error assessments also offer high-level views of system health and capabilities.
Impact on AI Safety and Effectiveness
The combination of customizable and pre-made evaluation tools on Galileo's platform significantly enhances AI system safety and effectiveness. These tools ensure systems not only complete tasks but also align with user goals and organizational values.
By enabling businesses to tailor evaluations to specific needs, Galileo helps ensure systems progress safely toward their objectives, reducing agentic operation error risks.
This streamlined evaluation process supports a proactive approach to AI safety, identifying and addressing potential issues early in deployment. As Sanyal points out, being able to "monitor the health of your agentic systems" substantially improves AI application maturity and reliability in precision-driven industries like healthcare and finance.
As AI agents become more prevalent across industries, evaluation and error management capabilities determine their reliability and utility. Customized frameworks and real-time monitoring help organizations use AI more precisely, supporting innovation and efficiency.
Galileo's agentic evaluations provide a comprehensive framework for monitoring and improving AI performance, enhancing safety and efficiency across applications. This improves user experience while ensuring AI developments align closely with business objectives.
Don't miss out on the full podcast conversation where Conor and Atindriyo dive deeper into these fascinating trends and reveal insights that could transform how you implement and evaluate agentic systems in your organization.
And check out other Chain of Thought episodes, where we discuss Generative AI for software engineers and AI leaders through stories, strategies, and practical techniques.
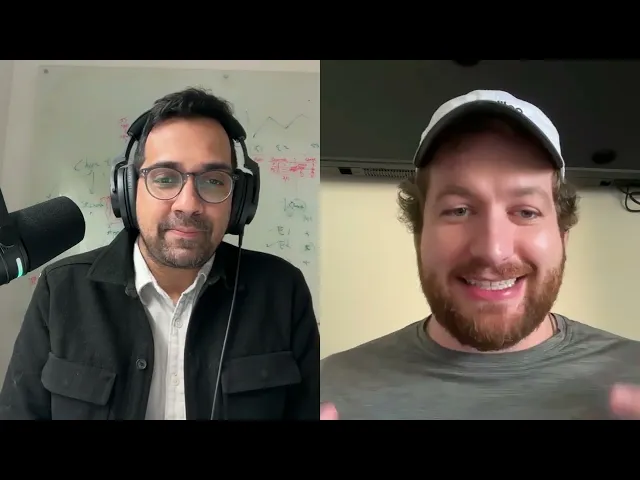
AI's agentic systems are making waves. Even Microsoft's Satya Nadella has shifted from talking about Software as a Service to "agents as a service." This isn't a minor update—it's reshaping how AI works across industries, opening doors to smarter automation and independent operations.
In a Chain of Thought podcast episode, Conor Bronsdon, Head of Developer Awareness at Galileo, and Atindriyo Sanyal, Galileo's CTO, explored agentic systems. They highlighted real-world impact, especially in customer service, where digital agents function as virtual team members.
Sanyal described how these systems combine large language model calls, database lookups, and more. "Agents get a little bit more complicated with additional components, which are stitched together as a directed acyclic graph or a DAG," he said. This architecture enables more intelligent interactions beyond simple Q&A.
The Structure and Functionality of Agentic Systems
Agentic systems represent a significant AI advancement. Unlike traditional single-task systems, they integrate multiple components to work independently. This evolution mirrors developments in Large Language Models (LLMs), databases, and APIs.

Key Components
As Sanyal explains, agentic systems have "a bunch of extra components that... get a little bit more complicated in terms of there's additional components, which are stitched together as, like, a directed acyclic graph or a DAG."
The directed acyclic graph structure is crucial to agentic systems, allowing sequential node operations—each potentially an LLM call, database lookup, or tool operation. This enables the system to function like a digital employee, handling tasks autonomously.
At their core, these systems rely on prompt engineering that guides the decision-making process. These prompts act as instruction sets, helping determine when to retrieve information from external sources versus generating responses from internal knowledge.
Memory components enable agents to recall past interactions and maintain context throughout complex conversations. This persistent memory creates more natural, human-like exchanges and allows for relationship-building between the AI and its users.
API integrations serve as the agent's connection to the external world, allowing it to perform actions like booking appointments, processing payments, or updating records in real-time. These capabilities transform agents from passive responders to active participants in business workflows.
Comparison With Traditional AI Systems
The design and purpose differences between traditional AI and agentic systems are stark. Traditional AI typically handles specific tasks with defined inputs and outputs, while agentic systems are built to mimic human decision-making across multiple tasks.
Traditional AI might handle one function at a time, but agentic systems combine several capabilities to reason and learn continuously, as highlighted in this AI frameworks comparison. This interconnection through the DAG structure helps these systems tackle complex challenges effectively. The choice between single vs multi-agent systems further influences their adaptability and performance.
Autonomy represents perhaps the most significant distinction. Traditional AI requires explicit human guidance for each new task, while agentic systems can determine the next steps independently based on context and goals, making them more versatile in unpredictable scenarios.
The cognitive architecture of agentic systems introduces a planning layer that allows for goal decomposition and prioritization. This enables agents to break complex objectives into manageable sub-tasks, much like a human professional would approach a multifaceted project.
Real-World Applications
Agentic systems show tremendous potential where automation and efficiency matter. For example, in financial services, they enhance customer service through chatbots that function as financial advisors, analyzing complex data for personalized guidance.
Bronsdon and Sanyal discussed the potential of a financial chatbot, asking, " Is the user's goal being met?" This goal-checking ability defines agentic systems. They satisfy client needs while maintaining security and compliance, critical in banking and healthcare. These systems adapt to real-time data changes, providing robust solutions for complex business challenges through advanced AI agentic workflows.
Agentic systems represent a stride toward smarter, self-sufficient AI applications, giving businesses better automation management tools. As this technology grows, it will continue transforming industries and driving digital advancement.
Evaluating Agentic Systems Through Task Completion and Error Management
As AI agents become increasingly integrated into business operations, performance evaluation becomes critical for reliability and effectiveness.
Importance of Task Completion Metrics
Agentic systems are autonomous, intelligent agents handling complex tasks. They go beyond responding to commands by utilizing databases, function calls, and logical operations to achieve goals. That's why task completion metrics are essential for evaluation.
"A notion of task completion or objective achievement” should be “one of the first things" examined when evaluating these systems, notes Sanyal. Whether it's a financial chatbot guiding investments or an AI agent processing data, measuring goal achievement is crucial. These metrics determine if agents are performing as intended, demonstrating their value and effectiveness.
Task completion metrics reveal alignment between user intent and agent action, highlighting instances where agents misinterpret requests. By benchmarking AI agents and tracking completion rates across different query types, organizations can identify pattern-based weaknesses requiring targeted improvements.
The granularity of these metrics matters significantly, as they should measure both overall task success and the accuracy of individual steps within multi-stage processes. This dual-layer assessment helps pinpoint exactly where breakdowns occur in complex workflows.
Customer satisfaction correlates strongly with successful task completion, making these metrics valuable business indicators beyond technical performance. When agents consistently achieve user goals efficiently, they build trust and encourage continued system engagement.
Error Compounding and its Implications
A major evaluation challenge is handling multiplying errors. Unlike traditional software, where isolated errors can be identified and fixed, agentic system mistakes can propagate. As Sanyal explains, "One mistake in any part of this workflow can lead to massive errors downstream."
Consider an AI system performing data retrieval through logical reasoning—an error anywhere can cascade through the system, amplifying its effects. Understanding error relationships helps teams develop better remediation and prevention strategies, strengthening system robustness and reliability.
The temporal dimension of error compounding presents unique challenges, as some errors remain dormant until triggered by specific conditions. These latent errors can create sudden system breakdowns when least expected, making comprehensive testing across diverse scenarios essential.
Recovery mechanisms become crucial design elements in agentic systems, providing failsafes that prevent complete system collapse when errors occur. Well-designed agents incorporate self-correction protocols that detect inconsistencies and attempt remediation before errors cascade.
Error correlation analysis offers valuable insights into systemic weaknesses, revealing whether failures cluster around particular components or interaction patterns. This analysis guides architectural improvements that strengthen not just error handling but the foundational design of the agent itself.
Galileo's Approach to Agentic Evaluations
Galileo leads in AI evaluation, particularly for agentic systems. Galileo’s approach addresses the growing need for robust tools that ensure AI systems perform well and align with intended goals.
Comprehensive Evaluation Platform
The complexity of agentic systems often renders traditional evaluation methods insufficient. New strategies have emerged, including real-time tracking, custom metrics, and detailed performance analysis. "There's no one size fits all," says Sanyal, highlighting the need for tailored solutions. This customization is crucial because AI applications vary greatly in components and goals.
Galileo's platform excels with its customizable evaluation metrics tailored to diverse agentic system needs. Sanyal continued, "We literally allow you to do two things. Number one, create your own agentic metric, which you care about… and apply it to the parts of the agents that you deem relevant."
A financial chatbot might use vector stores for data retrieval, requiring metrics focused on accuracy and relevance. Customization extends beyond creating metrics to integrating them into existing workflows, allowing developers to define metrics from simple Python functions to complex applications across their AI systems.
This flexibility lets developers evaluate precisely what matters most. Whether tracking an agent's goal-seeking steps or analyzing output accuracy, these custom metrics provide detailed performance insights. Monitoring tools like task completion metrics and error assessments also offer high-level views of system health and capabilities.
Impact on AI Safety and Effectiveness
The combination of customizable and pre-made evaluation tools on Galileo's platform significantly enhances AI system safety and effectiveness. These tools ensure systems not only complete tasks but also align with user goals and organizational values.
By enabling businesses to tailor evaluations to specific needs, Galileo helps ensure systems progress safely toward their objectives, reducing agentic operation error risks.
This streamlined evaluation process supports a proactive approach to AI safety, identifying and addressing potential issues early in deployment. As Sanyal points out, being able to "monitor the health of your agentic systems" substantially improves AI application maturity and reliability in precision-driven industries like healthcare and finance.
As AI agents become more prevalent across industries, evaluation and error management capabilities determine their reliability and utility. Customized frameworks and real-time monitoring help organizations use AI more precisely, supporting innovation and efficiency.
Galileo's agentic evaluations provide a comprehensive framework for monitoring and improving AI performance, enhancing safety and efficiency across applications. This improves user experience while ensuring AI developments align closely with business objectives.
Don't miss out on the full podcast conversation where Conor and Atindriyo dive deeper into these fascinating trends and reveal insights that could transform how you implement and evaluate agentic systems in your organization.
And check out other Chain of Thought episodes, where we discuss Generative AI for software engineers and AI leaders through stories, strategies, and practical techniques.
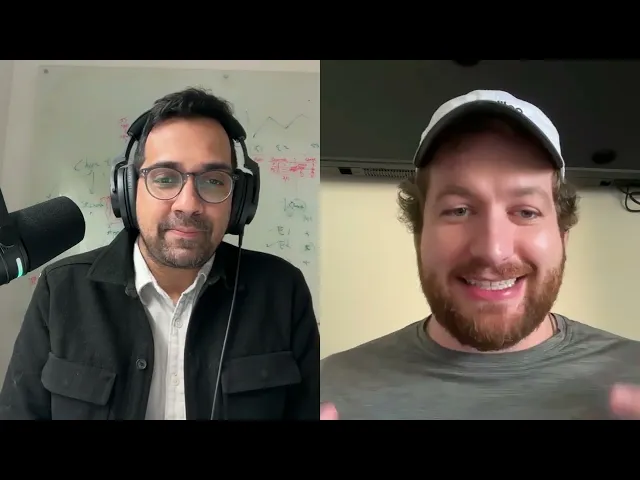
AI's agentic systems are making waves. Even Microsoft's Satya Nadella has shifted from talking about Software as a Service to "agents as a service." This isn't a minor update—it's reshaping how AI works across industries, opening doors to smarter automation and independent operations.
In a Chain of Thought podcast episode, Conor Bronsdon, Head of Developer Awareness at Galileo, and Atindriyo Sanyal, Galileo's CTO, explored agentic systems. They highlighted real-world impact, especially in customer service, where digital agents function as virtual team members.
Sanyal described how these systems combine large language model calls, database lookups, and more. "Agents get a little bit more complicated with additional components, which are stitched together as a directed acyclic graph or a DAG," he said. This architecture enables more intelligent interactions beyond simple Q&A.
The Structure and Functionality of Agentic Systems
Agentic systems represent a significant AI advancement. Unlike traditional single-task systems, they integrate multiple components to work independently. This evolution mirrors developments in Large Language Models (LLMs), databases, and APIs.

Key Components
As Sanyal explains, agentic systems have "a bunch of extra components that... get a little bit more complicated in terms of there's additional components, which are stitched together as, like, a directed acyclic graph or a DAG."
The directed acyclic graph structure is crucial to agentic systems, allowing sequential node operations—each potentially an LLM call, database lookup, or tool operation. This enables the system to function like a digital employee, handling tasks autonomously.
At their core, these systems rely on prompt engineering that guides the decision-making process. These prompts act as instruction sets, helping determine when to retrieve information from external sources versus generating responses from internal knowledge.
Memory components enable agents to recall past interactions and maintain context throughout complex conversations. This persistent memory creates more natural, human-like exchanges and allows for relationship-building between the AI and its users.
API integrations serve as the agent's connection to the external world, allowing it to perform actions like booking appointments, processing payments, or updating records in real-time. These capabilities transform agents from passive responders to active participants in business workflows.
Comparison With Traditional AI Systems
The design and purpose differences between traditional AI and agentic systems are stark. Traditional AI typically handles specific tasks with defined inputs and outputs, while agentic systems are built to mimic human decision-making across multiple tasks.
Traditional AI might handle one function at a time, but agentic systems combine several capabilities to reason and learn continuously, as highlighted in this AI frameworks comparison. This interconnection through the DAG structure helps these systems tackle complex challenges effectively. The choice between single vs multi-agent systems further influences their adaptability and performance.
Autonomy represents perhaps the most significant distinction. Traditional AI requires explicit human guidance for each new task, while agentic systems can determine the next steps independently based on context and goals, making them more versatile in unpredictable scenarios.
The cognitive architecture of agentic systems introduces a planning layer that allows for goal decomposition and prioritization. This enables agents to break complex objectives into manageable sub-tasks, much like a human professional would approach a multifaceted project.
Real-World Applications
Agentic systems show tremendous potential where automation and efficiency matter. For example, in financial services, they enhance customer service through chatbots that function as financial advisors, analyzing complex data for personalized guidance.
Bronsdon and Sanyal discussed the potential of a financial chatbot, asking, " Is the user's goal being met?" This goal-checking ability defines agentic systems. They satisfy client needs while maintaining security and compliance, critical in banking and healthcare. These systems adapt to real-time data changes, providing robust solutions for complex business challenges through advanced AI agentic workflows.
Agentic systems represent a stride toward smarter, self-sufficient AI applications, giving businesses better automation management tools. As this technology grows, it will continue transforming industries and driving digital advancement.
Evaluating Agentic Systems Through Task Completion and Error Management
As AI agents become increasingly integrated into business operations, performance evaluation becomes critical for reliability and effectiveness.
Importance of Task Completion Metrics
Agentic systems are autonomous, intelligent agents handling complex tasks. They go beyond responding to commands by utilizing databases, function calls, and logical operations to achieve goals. That's why task completion metrics are essential for evaluation.
"A notion of task completion or objective achievement” should be “one of the first things" examined when evaluating these systems, notes Sanyal. Whether it's a financial chatbot guiding investments or an AI agent processing data, measuring goal achievement is crucial. These metrics determine if agents are performing as intended, demonstrating their value and effectiveness.
Task completion metrics reveal alignment between user intent and agent action, highlighting instances where agents misinterpret requests. By benchmarking AI agents and tracking completion rates across different query types, organizations can identify pattern-based weaknesses requiring targeted improvements.
The granularity of these metrics matters significantly, as they should measure both overall task success and the accuracy of individual steps within multi-stage processes. This dual-layer assessment helps pinpoint exactly where breakdowns occur in complex workflows.
Customer satisfaction correlates strongly with successful task completion, making these metrics valuable business indicators beyond technical performance. When agents consistently achieve user goals efficiently, they build trust and encourage continued system engagement.
Error Compounding and its Implications
A major evaluation challenge is handling multiplying errors. Unlike traditional software, where isolated errors can be identified and fixed, agentic system mistakes can propagate. As Sanyal explains, "One mistake in any part of this workflow can lead to massive errors downstream."
Consider an AI system performing data retrieval through logical reasoning—an error anywhere can cascade through the system, amplifying its effects. Understanding error relationships helps teams develop better remediation and prevention strategies, strengthening system robustness and reliability.
The temporal dimension of error compounding presents unique challenges, as some errors remain dormant until triggered by specific conditions. These latent errors can create sudden system breakdowns when least expected, making comprehensive testing across diverse scenarios essential.
Recovery mechanisms become crucial design elements in agentic systems, providing failsafes that prevent complete system collapse when errors occur. Well-designed agents incorporate self-correction protocols that detect inconsistencies and attempt remediation before errors cascade.
Error correlation analysis offers valuable insights into systemic weaknesses, revealing whether failures cluster around particular components or interaction patterns. This analysis guides architectural improvements that strengthen not just error handling but the foundational design of the agent itself.
Galileo's Approach to Agentic Evaluations
Galileo leads in AI evaluation, particularly for agentic systems. Galileo’s approach addresses the growing need for robust tools that ensure AI systems perform well and align with intended goals.
Comprehensive Evaluation Platform
The complexity of agentic systems often renders traditional evaluation methods insufficient. New strategies have emerged, including real-time tracking, custom metrics, and detailed performance analysis. "There's no one size fits all," says Sanyal, highlighting the need for tailored solutions. This customization is crucial because AI applications vary greatly in components and goals.
Galileo's platform excels with its customizable evaluation metrics tailored to diverse agentic system needs. Sanyal continued, "We literally allow you to do two things. Number one, create your own agentic metric, which you care about… and apply it to the parts of the agents that you deem relevant."
A financial chatbot might use vector stores for data retrieval, requiring metrics focused on accuracy and relevance. Customization extends beyond creating metrics to integrating them into existing workflows, allowing developers to define metrics from simple Python functions to complex applications across their AI systems.
This flexibility lets developers evaluate precisely what matters most. Whether tracking an agent's goal-seeking steps or analyzing output accuracy, these custom metrics provide detailed performance insights. Monitoring tools like task completion metrics and error assessments also offer high-level views of system health and capabilities.
Impact on AI Safety and Effectiveness
The combination of customizable and pre-made evaluation tools on Galileo's platform significantly enhances AI system safety and effectiveness. These tools ensure systems not only complete tasks but also align with user goals and organizational values.
By enabling businesses to tailor evaluations to specific needs, Galileo helps ensure systems progress safely toward their objectives, reducing agentic operation error risks.
This streamlined evaluation process supports a proactive approach to AI safety, identifying and addressing potential issues early in deployment. As Sanyal points out, being able to "monitor the health of your agentic systems" substantially improves AI application maturity and reliability in precision-driven industries like healthcare and finance.
As AI agents become more prevalent across industries, evaluation and error management capabilities determine their reliability and utility. Customized frameworks and real-time monitoring help organizations use AI more precisely, supporting innovation and efficiency.
Galileo's agentic evaluations provide a comprehensive framework for monitoring and improving AI performance, enhancing safety and efficiency across applications. This improves user experience while ensuring AI developments align closely with business objectives.
Don't miss out on the full podcast conversation where Conor and Atindriyo dive deeper into these fascinating trends and reveal insights that could transform how you implement and evaluate agentic systems in your organization.
And check out other Chain of Thought episodes, where we discuss Generative AI for software engineers and AI leaders through stories, strategies, and practical techniques.
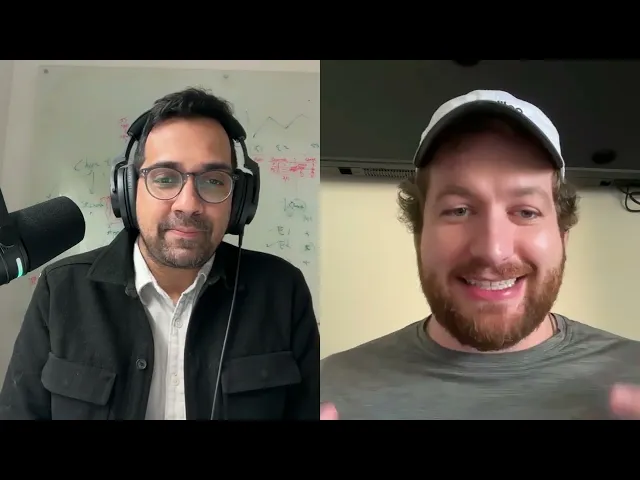
AI's agentic systems are making waves. Even Microsoft's Satya Nadella has shifted from talking about Software as a Service to "agents as a service." This isn't a minor update—it's reshaping how AI works across industries, opening doors to smarter automation and independent operations.
In a Chain of Thought podcast episode, Conor Bronsdon, Head of Developer Awareness at Galileo, and Atindriyo Sanyal, Galileo's CTO, explored agentic systems. They highlighted real-world impact, especially in customer service, where digital agents function as virtual team members.
Sanyal described how these systems combine large language model calls, database lookups, and more. "Agents get a little bit more complicated with additional components, which are stitched together as a directed acyclic graph or a DAG," he said. This architecture enables more intelligent interactions beyond simple Q&A.
The Structure and Functionality of Agentic Systems
Agentic systems represent a significant AI advancement. Unlike traditional single-task systems, they integrate multiple components to work independently. This evolution mirrors developments in Large Language Models (LLMs), databases, and APIs.

Key Components
As Sanyal explains, agentic systems have "a bunch of extra components that... get a little bit more complicated in terms of there's additional components, which are stitched together as, like, a directed acyclic graph or a DAG."
The directed acyclic graph structure is crucial to agentic systems, allowing sequential node operations—each potentially an LLM call, database lookup, or tool operation. This enables the system to function like a digital employee, handling tasks autonomously.
At their core, these systems rely on prompt engineering that guides the decision-making process. These prompts act as instruction sets, helping determine when to retrieve information from external sources versus generating responses from internal knowledge.
Memory components enable agents to recall past interactions and maintain context throughout complex conversations. This persistent memory creates more natural, human-like exchanges and allows for relationship-building between the AI and its users.
API integrations serve as the agent's connection to the external world, allowing it to perform actions like booking appointments, processing payments, or updating records in real-time. These capabilities transform agents from passive responders to active participants in business workflows.
Comparison With Traditional AI Systems
The design and purpose differences between traditional AI and agentic systems are stark. Traditional AI typically handles specific tasks with defined inputs and outputs, while agentic systems are built to mimic human decision-making across multiple tasks.
Traditional AI might handle one function at a time, but agentic systems combine several capabilities to reason and learn continuously, as highlighted in this AI frameworks comparison. This interconnection through the DAG structure helps these systems tackle complex challenges effectively. The choice between single vs multi-agent systems further influences their adaptability and performance.
Autonomy represents perhaps the most significant distinction. Traditional AI requires explicit human guidance for each new task, while agentic systems can determine the next steps independently based on context and goals, making them more versatile in unpredictable scenarios.
The cognitive architecture of agentic systems introduces a planning layer that allows for goal decomposition and prioritization. This enables agents to break complex objectives into manageable sub-tasks, much like a human professional would approach a multifaceted project.
Real-World Applications
Agentic systems show tremendous potential where automation and efficiency matter. For example, in financial services, they enhance customer service through chatbots that function as financial advisors, analyzing complex data for personalized guidance.
Bronsdon and Sanyal discussed the potential of a financial chatbot, asking, " Is the user's goal being met?" This goal-checking ability defines agentic systems. They satisfy client needs while maintaining security and compliance, critical in banking and healthcare. These systems adapt to real-time data changes, providing robust solutions for complex business challenges through advanced AI agentic workflows.
Agentic systems represent a stride toward smarter, self-sufficient AI applications, giving businesses better automation management tools. As this technology grows, it will continue transforming industries and driving digital advancement.
Evaluating Agentic Systems Through Task Completion and Error Management
As AI agents become increasingly integrated into business operations, performance evaluation becomes critical for reliability and effectiveness.
Importance of Task Completion Metrics
Agentic systems are autonomous, intelligent agents handling complex tasks. They go beyond responding to commands by utilizing databases, function calls, and logical operations to achieve goals. That's why task completion metrics are essential for evaluation.
"A notion of task completion or objective achievement” should be “one of the first things" examined when evaluating these systems, notes Sanyal. Whether it's a financial chatbot guiding investments or an AI agent processing data, measuring goal achievement is crucial. These metrics determine if agents are performing as intended, demonstrating their value and effectiveness.
Task completion metrics reveal alignment between user intent and agent action, highlighting instances where agents misinterpret requests. By benchmarking AI agents and tracking completion rates across different query types, organizations can identify pattern-based weaknesses requiring targeted improvements.
The granularity of these metrics matters significantly, as they should measure both overall task success and the accuracy of individual steps within multi-stage processes. This dual-layer assessment helps pinpoint exactly where breakdowns occur in complex workflows.
Customer satisfaction correlates strongly with successful task completion, making these metrics valuable business indicators beyond technical performance. When agents consistently achieve user goals efficiently, they build trust and encourage continued system engagement.
Error Compounding and its Implications
A major evaluation challenge is handling multiplying errors. Unlike traditional software, where isolated errors can be identified and fixed, agentic system mistakes can propagate. As Sanyal explains, "One mistake in any part of this workflow can lead to massive errors downstream."
Consider an AI system performing data retrieval through logical reasoning—an error anywhere can cascade through the system, amplifying its effects. Understanding error relationships helps teams develop better remediation and prevention strategies, strengthening system robustness and reliability.
The temporal dimension of error compounding presents unique challenges, as some errors remain dormant until triggered by specific conditions. These latent errors can create sudden system breakdowns when least expected, making comprehensive testing across diverse scenarios essential.
Recovery mechanisms become crucial design elements in agentic systems, providing failsafes that prevent complete system collapse when errors occur. Well-designed agents incorporate self-correction protocols that detect inconsistencies and attempt remediation before errors cascade.
Error correlation analysis offers valuable insights into systemic weaknesses, revealing whether failures cluster around particular components or interaction patterns. This analysis guides architectural improvements that strengthen not just error handling but the foundational design of the agent itself.
Galileo's Approach to Agentic Evaluations
Galileo leads in AI evaluation, particularly for agentic systems. Galileo’s approach addresses the growing need for robust tools that ensure AI systems perform well and align with intended goals.
Comprehensive Evaluation Platform
The complexity of agentic systems often renders traditional evaluation methods insufficient. New strategies have emerged, including real-time tracking, custom metrics, and detailed performance analysis. "There's no one size fits all," says Sanyal, highlighting the need for tailored solutions. This customization is crucial because AI applications vary greatly in components and goals.
Galileo's platform excels with its customizable evaluation metrics tailored to diverse agentic system needs. Sanyal continued, "We literally allow you to do two things. Number one, create your own agentic metric, which you care about… and apply it to the parts of the agents that you deem relevant."
A financial chatbot might use vector stores for data retrieval, requiring metrics focused on accuracy and relevance. Customization extends beyond creating metrics to integrating them into existing workflows, allowing developers to define metrics from simple Python functions to complex applications across their AI systems.
This flexibility lets developers evaluate precisely what matters most. Whether tracking an agent's goal-seeking steps or analyzing output accuracy, these custom metrics provide detailed performance insights. Monitoring tools like task completion metrics and error assessments also offer high-level views of system health and capabilities.
Impact on AI Safety and Effectiveness
The combination of customizable and pre-made evaluation tools on Galileo's platform significantly enhances AI system safety and effectiveness. These tools ensure systems not only complete tasks but also align with user goals and organizational values.
By enabling businesses to tailor evaluations to specific needs, Galileo helps ensure systems progress safely toward their objectives, reducing agentic operation error risks.
This streamlined evaluation process supports a proactive approach to AI safety, identifying and addressing potential issues early in deployment. As Sanyal points out, being able to "monitor the health of your agentic systems" substantially improves AI application maturity and reliability in precision-driven industries like healthcare and finance.
As AI agents become more prevalent across industries, evaluation and error management capabilities determine their reliability and utility. Customized frameworks and real-time monitoring help organizations use AI more precisely, supporting innovation and efficiency.
Galileo's agentic evaluations provide a comprehensive framework for monitoring and improving AI performance, enhancing safety and efficiency across applications. This improves user experience while ensuring AI developments align closely with business objectives.
Don't miss out on the full podcast conversation where Conor and Atindriyo dive deeper into these fascinating trends and reveal insights that could transform how you implement and evaluate agentic systems in your organization.
And check out other Chain of Thought episodes, where we discuss Generative AI for software engineers and AI leaders through stories, strategies, and practical techniques.
Conor Bronsdon
Conor Bronsdon
Conor Bronsdon
Conor Bronsdon