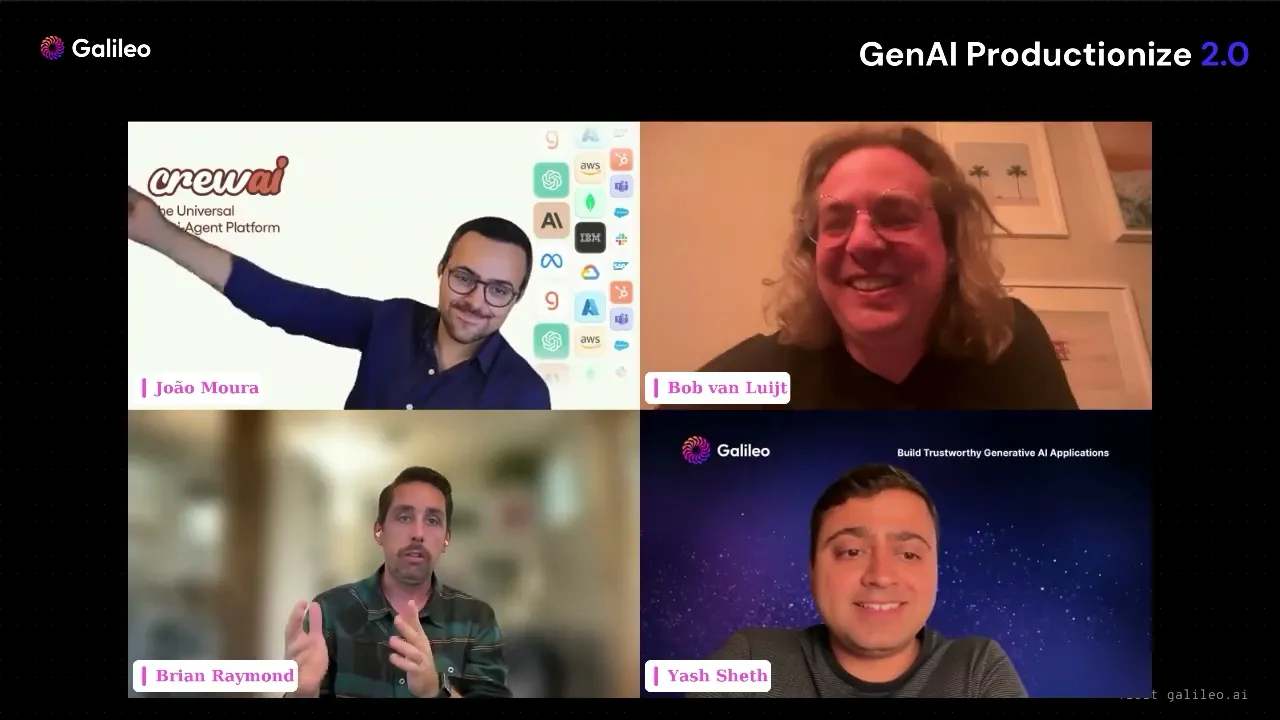
Apr 8, 2025
How to Detect Coordinated Attacks in Multi-Agent AI Systems

Conor Bronsdon
Head of Developer Awareness

Multi-agent AI architectures—where multiple AI systems collaborate to solve complex problems—create rich new attack surfaces. Their distributed decision-making and agent-to-agent dependencies introduce vulnerabilities that single-agent systems simply don't face, requiring specialized security approaches.
The stakes are particularly high in enterprise settings. Compromised financial systems face market manipulation. Healthcare networks risk patient data exposure. Manufacturing systems could sabotage production quality.
This article explores the coordinated threat landscape for multi-agent AI systems, examines common attack vectors, and shares practical strategies for detecting and preventing these threats.
We recently explored this topic on our Chain of Thought podcast, where industry experts shared practical insights and real-world implementation strategies.
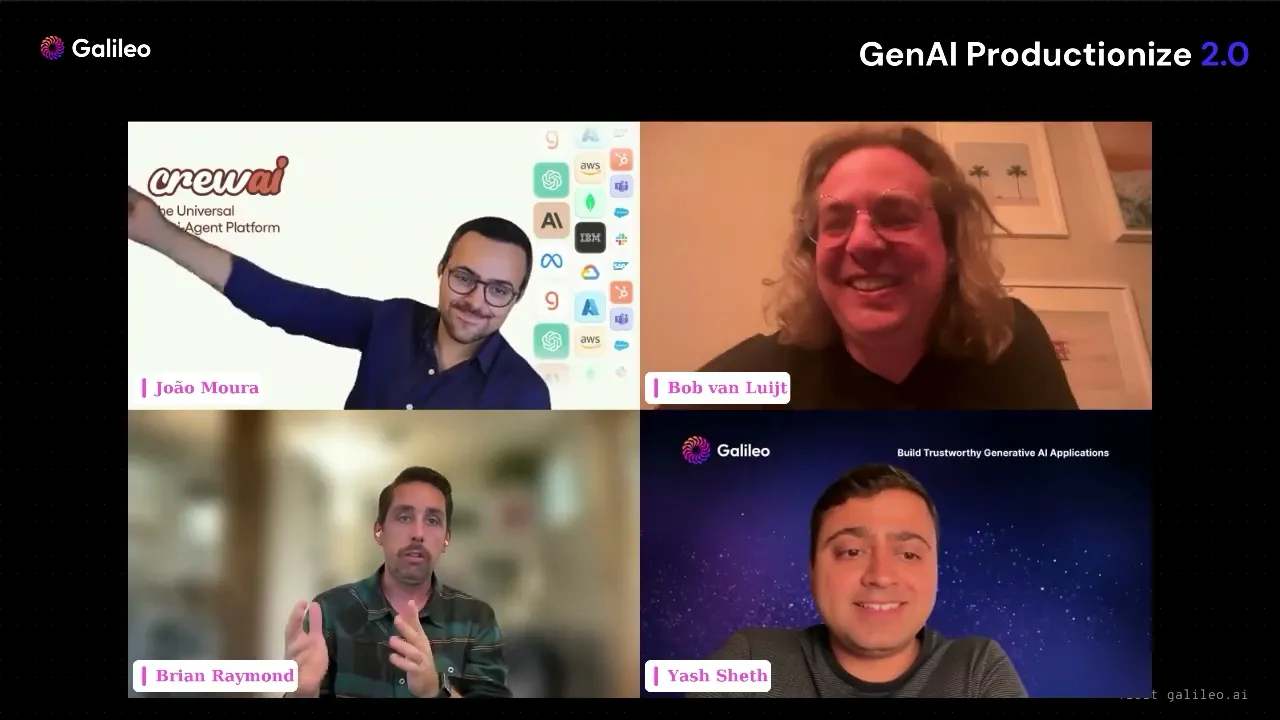
Six Multi-Agent Attack Vectors
Multi-agent AI architectures introduce unique security challenges beyond those found in single-agent deployments. The distributed nature of these systems creates specialized attack surfaces that malicious actors can exploit. Here are the primary coordinated attack vectors threatening multi-agent AI systems:
Trust Exploitation Between Agents: Attackers manipulate the trust relationships that form the foundation of agent cooperation. By compromising a trusted agent, attackers can issue commands that propagate unchallenged through the network. These "confused deputy" attacks succeed because agents often implicitly trust inputs from other agents in their ecosystem without sufficient verification mechanisms.
Control-Flow Hijacking: These attacks exploit vulnerabilities in metadata transmission between agents to reroute invocations and execute malicious code. Studies have shown success rates as high as 100% in certain multi-agent configurations, allowing attackers to turn legitimate agents into unwitting accomplices.
Message Interception and Corruption: By positioning themselves in communication pathways between agents, attackers can intercept, modify, or fabricate messages. This compromises the integrity of inter-agent communication, leading to corrupted decisions based on tampered information, particularly devastating in systems like autonomous vehicle fleets.
Goal Manipulation: This involves altering the objectives of distributed agents by manipulating their decision-making inputs. Agents can be subtly redirected toward harmful goals while appearing to operate normally. This is particularly effective in financial systems where automated trading agents can be steered toward destabilizing market activities.
Byzantine Behavior Patterns: Attackers introduce malicious or faulty agents that behave erratically or inconsistently, preventing the system from reaching consensus. In decentralized systems like energy grids, these attacks can cause cascading failures in load distribution, leading to widespread outages.
Sybil Attacks: By creating multiple fake identities within the system, attackers gain disproportionate influence over decision-making processes. These attacks are particularly effective against voting-based consensus mechanisms, allowing attackers to overwhelm legitimate agents with fabricated identities.
These attack vectors rarely appear in isolation. In sophisticated attack scenarios, they combine to create devastating effects that are difficult to detect and mitigate.
For example, an attacker might begin with a Sybil attack to establish multiple identities within a system, and then use those identities to conduct Byzantine behaviors that disrupt consensus mechanisms while simultaneously exploiting trust relationships to propagate malicious commands.

Detection Strategies for Coordinated Attacks in Multi-Agent AI
Detecting coordinated attacks in multi-agent AI demands interconnected defense strategies.
Implement Advanced Behavioral Analysis for Agent Anomalies
Behavioral analysis forms the foundation of any robust multi-agent security system. To effectively implement this approach, start by collecting comprehensive baselines of normal agent behavior across multiple dimensions: resource utilization, communication patterns, decision-making sequences, and goal achievement rates.
Your anomaly detection models should incorporate both unsupervised and supervised techniques. Use clustering algorithms to identify behavioral outliers and deep learning models to detect subtle deviations from established patterns. Frameworks like PyTorch and TensorFlow provide the necessary tools for building these complex detection models with minimal overhead.
Focus your monitoring on key behavioral indicators: sudden changes in communication frequency, abnormal resource consumption spikes, unexplained goal deviations, and altered interaction patterns with external systems. These metrics have proven especially effective at identifying early stages of agent compromise before attacks fully materialize.
Time-series analysis of agent behaviors provides a critical context for anomaly detection. Implement sliding window algorithms that can detect gradual behavioral shifts, which might indicate sophisticated attacks unfolding over extended periods. This approach significantly reduces false positives while maintaining detection sensitivity.
Incorporate feedback loops that continuously improve anomaly detection accuracy. When potential anomalies are detected, human security analysts should review and classify them, with these classifications feeding back into model training. This human-in-the-loop approach dramatically improves detection accuracy while reducing alert fatigue among security teams.
Deploy Inter-Agent Communication Monitoring Systems
Communication between agents represents a critical attack surface in multi-agent systems. Implement secure message passing protocols with end-to-end encryption and integrity verification to establish a foundation for secure agent interactions. This ensures that monitored messages haven't been tampered with during transmission.
Deploy network traffic analysis tools that examine communication patterns rather than just content. Graph-based approaches are particularly effective, allowing you to model the communication network and identify abnormal structures or unexpected relationships between agents. Sudden changes in communication graph density or clustering coefficients often indicate coordinated attacks in progress.
Temporal analysis of message exchanges reveals sophisticated attack patterns that static analysis might miss. Implement sequential pattern mining to identify suspicious communication sequences, and use entropy-based measurements to detect information leakage or covert channels between agents. These techniques excel at uncovering hidden command-and-control communications in compromised systems.
When designing monitoring systems, balance detection capabilities against performance impact. Passive monitoring approaches that analyze communication metadata rather than message content provide efficient broad coverage, while targeted deep inspection can be selectively applied to suspicious exchanges. This tiered approach maintains system performance while providing comprehensive security.
For high-throughput environments, consider distributed monitoring architectures that scale horizontally with your agent population. Leverage open standards like MQTT for messaging and implement message sampling techniques that maintain security while reducing computational overhead. This approach ensures monitoring effectiveness even as your multi-agent system grows.
Establish Distributed Trust Verification Frameworks
Distributed trust verification provides a critical security layer for detecting and preventing coordinated attacks in multi-agent AI systems. Implement Byzantine fault tolerance mechanisms that allow your system to function correctly even when some agents behave maliciously or fail unexpectedly. Practical Byzantine Fault Tolerance (PBFT) and Federated Byzantine Agreement (FBA) algorithms offer established approaches for maintaining consensus in hostile environments.
Develop reputation systems that continuously evaluate agent trustworthiness based on past behavior, communication patterns, and task outcomes. These systems should incorporate temporal decay factors that give greater weight to recent actions while maintaining historical context. This prevents compromised agents from leveraging previously established trust to conduct attacks.
Zero-knowledge proofs enable secure agent verification without exposing sensitive information or credentials. Implement these cryptographic protocols to verify agent identity and state without revealing the underlying data, dramatically reducing the attack surface. This approach is particularly valuable for verifying agent integrity without compromising system performance.
Consider implementing the MAESTRO Framework to address agent-specific threats through layered security and continuous adaptation. This framework provides comprehensive coverage against evolving attack vectors while maintaining operational effectiveness in dynamic environments.
For systems requiring maximum resilience, deploy Moving Target Defense strategies that dynamically alter system configurations and trust relationships. By regularly changing trust verification parameters, communication protocols, and security thresholds, you create an environment where attackers cannot rely on static system properties.
This approach significantly increases the cost and complexity of mounting successful coordinated attacks. By comprehensively addressing the threats in multi-agent decision-making, organizations can enhance their detection capabilities against coordinated attacks in multi-agent AI systems.

Prevention Mechanisms and Safeguards for Coordinated Attacks in Multi-Agent AI
Let’s examine how organizations can significantly reduce their vulnerability to coordinated attacks while maintaining system functionality.
Design Secure Multi-Agent Architectures from the Ground Up
Defense-in-depth strategies and adherence to AI security best practices are essential when building multi-agent systems. Each agent should operate with clearly defined boundaries and explicitly granted permissions following the principle of least privilege. This foundational approach minimizes the attack surface exposed to potential adversaries.
Implement secure communication channels between agents using strong encryption and message verification. This prevents attackers from intercepting or tampering with inter-agent communication, which could otherwise lead to compromised operations or data exfiltration.
Component isolation is particularly effective in preventing control-flow hijacking attacks. Consider containerization technologies to enforce this isolation—frameworks like AutoGen leverage Docker environments to securely delegate tasks between agents.
Trust boundaries should be explicitly defined and enforced between agents that operate at different privilege levels or handle varying sensitivity of data. This compartmentalization prevents compromise of one agent from cascading throughout the entire system.
Formal verification methods also validate agent interactions against security policies before deployment. This proactive approach catches potential vulnerabilities during development rather than after exploitation, significantly reducing remediation costs and security risks.
Implement Advanced Authentication and Authorization Controls
Zero-trust architectures should form the foundation of your authentication strategy for multi-agent systems. Never implicitly trust any agent, even those operating within your network perimeter. Instead, verify each agent's identity and authorization status for every operation.
Implement mutual TLS (mTLS) between agents to ensure bidirectional authentication. This prevents attackers from impersonating legitimate agents and hijacking trusted communication channels. Regular certificate rotation further reduces the risk of compromised credentials being exploited.
Role-based access controls (RBAC) should dynamically adjust to changing system states and agent responsibilities. Frameworks like CrewAI offer specialized RBAC implementations designed specifically for multi-agent environments, with human oversight capabilities to maintain security guardrails.
In addition, implement cryptographic identity verification using asymmetric key pairs assigned to each agent. This provides strong authentication guarantees while enabling secure message signing and verification between agents. Consider hardware security modules for storing critical agent credentials in high-security environments.
Authentication attestation chains create a verifiable proof of an agent's identity and integrity, ensuring that only properly configured and uncompromised agents can participate in system operations. This is particularly valuable in distributed environments where agents may operate across multiple trust domains.
Monitor Your Multi-Agent AI Systems with Galileo
Detecting and preventing coordinated attacks in multi-agent AI requires a sophisticated approach that detects threats across multiple agents, monitors communication channels, and prevents coordinated attacks before they compromise your systems.
Galileo delivers a robust solution for monitoring multi-agent AI systems with comprehensive capabilities designed specifically for these distributed environments:
Real-time Communication Monitoring: Galileo continuously monitors inter-agent communications to detect anomalies, suspicious patterns, and potential tampering attempts.
Agent Authentication Framework: Galileo implements strong identity verification mechanisms to prevent spoofing and ensure that every interaction occurs between trusted agents.
Distributed Threat Intelligence: Galileo collects and analyzes signals across your entire agent ecosystem, enabling early detection of coordinated attacks that might otherwise go unnoticed when examined in isolation.
Chain-of-Thought Protection: Galileo’s advanced sandboxing and validation tools prevent malicious inputs from propagating through agent chains, protecting against prompt injection attacks where compromised outputs from one agent become dangerous inputs for others.
Centralized Security Governance: Galileo provides unified security policies and controls across your entire multi-agent system, ensuring consistent protection regardless of where agents are deployed or how they interact with each other and external resources.
Get started with Galileo today to monitor your multi-agent AI systems and build more reliable, effective, and trustworthy AI applications.
Conor Bronsdon